Machine Learning at the University of Toronto
The Department of Computer Science at the University of Toronto has several faculty members working in the area of machine learning, neural networks, statistical pattern recognition, probabilistic planning, and adaptive systems. In addition, many faculty members inside and outside the department whose primary research interests are in other areas have specific research projects involving machine learning in some way.
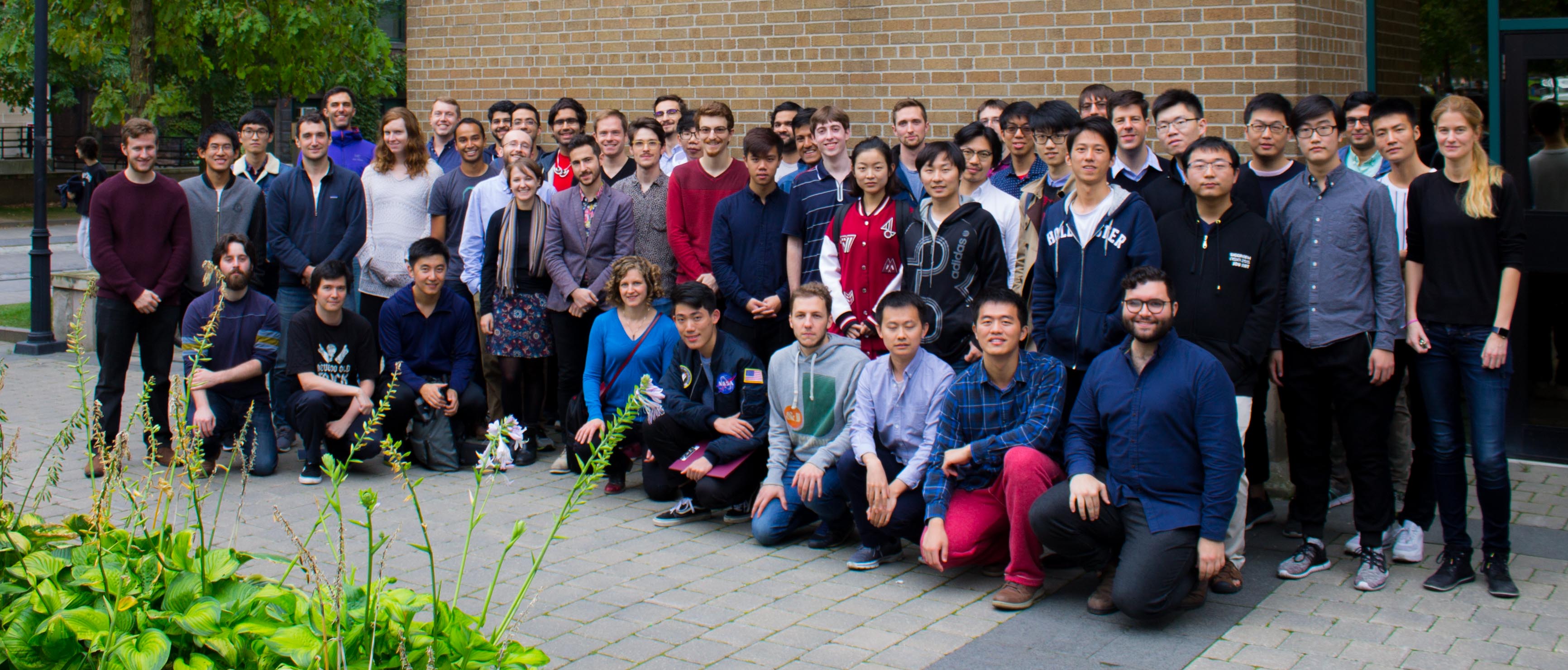